Dana Nau
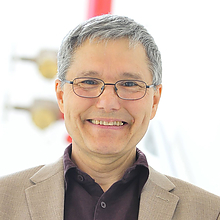
Dana Nau is a professor in the Department of Computer Science and the Institute for Systems Research.
His research focuses on artificial intelligence (AI), especially in the areas of game theory and AI planning. Nau also co-directs the Laboratory for Computational Cultural Dynamics.
He is a fellow of AAAI, and has received dozens of other awards and honors.
Some of Nau's best-known accomplishments include (1) discovering game-tree pathology, which has led to subsequent research by dozens of other researchers over the past three decades; (2) co-authoring the strategy-generation algorithm that enabled the Bridge Baron program to win the 1997 world championship of computer bridge; (3) leading the development of the SHOP and SHOP2 automated-planning systems, which have been used in many hundreds of projects in industry, government, and academia; (4) co-authoring a widely used graduate-level textbook, "Automated Planning: Theory and Practice."
He received his doctorate in computer science from Duke University in 1979.
Publications
2011
2011. Temporal Visualization of Social Network Dynamics: Prototypes for Nation of Neighbors. Social Computing, Behavioral-Cultural Modeling and PredictionSocial Computing, Behavioral-Cultural Modeling and Prediction. 6589:309-316.
2011. Speeding Up Network Layout and Centrality Measures for Social Computing Goals. Social Computing, Behavioral-Cultural Modeling and PredictionSocial Computing, Behavioral-Cultural Modeling and Prediction. 6589:244-251.
2010
2010. Emergence of cooperative societies in evolutionary games. :1793-1800.
2010. Evolution of state-dependent risk preferences. ACM Transactions on Intelligent Systems and Technology (TIST). 1(1):6:1–6:21-6:1–6:21.
2010. When is it better not to look ahead? Artificial Intelligence. 174(16–17):1323-1338.
2009
2009. Task decomposition on abstract states, for planning under nondeterminism. Artificial Intelligence. 173(5–6):669-695.
2009. Artificial Intelligence and Automation. Springer Handbook of Automation. :249-268.
2009. Within epsilon of optimal play in the cultaptation social learning game. :1327-1328.
2009. Planning for Interactions among Autonomous Agents. Programming Multi-Agent Systems. 5442:1-23.
2008
2008. Synthesis of strategies from interaction traces. :855-862.
2008. Computational Cultural Dynamics. Intelligent Systems, IEEE. 23(4):18-19.
2007
2007. Current Trends in Automated Planning. AI Magazine. 28(4):43-43.
2007. Probabilistic go theories.
2007. Finding Most Probable Worlds of Probabilistic Logic Programs. Scalable Uncertainty Management. 4772:45-59.
2007. CARA: A Cultural-Reasoning Architecture. IEEE Intelligent Systems. 22(2):12-16.
2007. Finding most probable worlds of probabilistic logic programs. Scalable Uncertainty Management. :45-59.
2007. Computing most probable worlds of action probabilistic logic programs: scalable estimation for 10^30,000 worlds. Annals of Mathematics and Artificial Intelligence. 51(2):295-331.
2006
2006. The incompleteness of planning with volatile external information. FRONTIERS IN ARTIFICIAL IN℡LIGENCE AND APPLICATIONS. 141:839-839.
2006. Maintaining cooperation in noisy environments. 21:1561-1561.
2006. Learning to do HTN planning. :390-393.
2006. A stochastic language for modelling opponent agents. Proceedings of the fifth international joint conference on Autonomous agents and multiagent systems. :244-246.
2005
2005. A motion closed world asumption. 19:621-621.
2005. A hierarchical task-network planner based on symbolic model checking. :300-309.
2005. Web Service Composition with Volatile Information. The Semantic Web – ISWC 2005. 3729:52-66.
2005. Game-tree search with combinatorially large belief states. 19:254-254.
2005. Applications of SHOP and SHOP2. Intelligent Systems, IEEE. 20(2):34-41.
2005. Information gathering during planning for Web Service composition. Web Semantics: Science, Services and Agents on the World Wide Web. 3(2–3):183-205.
2005. Using domain-configurable search control for probabilistic planning. 20:1169-1169.
2005. Learning approximate preconditions for methods in hierarchical plans. :337-344.
2005. Learning preconditions for planning from plan traces and HTN structure. Computational Intelligence. 21(4):388-413.
2005. Going far, logically. 19:615-615.
2004
2004. Forward-chaining planning in nondeterministic domains. :513-518.
2004. HTN planning for Web Service composition using SHOP2. Web Semantics: Science, Services and Agents on the World Wide Web. 1(4):377-396.
2004. Information Gathering During Planning for Web Service Composition. The Semantic Web – ISWC 2004. 3298:335-349.
2004. A logic of motion. :85-94.
2004. Utilizing volatile external information during planning. Proceedings of the European Conference on Artificial Intelligence (ECAI). :647-651.
2004. Plan Databases: Model and Algebra. Foundations of Information and Knowledge Systems. 2942:302-319.
2003
2003. Planning in Answer Set Programming Using Ordered Task Decomposition. KI 2003: Advances in Artificial Intelligence. 2821:490-504.
2003. SHOP2: An HTN planning system. J. Artif. Intell. Res. (JAIR). 20:379-404.
2003. IMPACTing SHOP: Putting an AI Planner Into a Multi-Agent Environment. Annals of Mathematics and Artificial Intelligence. 37(4):381-407.
2003. Algorithms for selecting cutters in multi-part milling problems. Computer-Aided Design. 35(9):825-839.
2003. Automating DAML-S Web Services Composition Using SHOP2. The Semantic Web - ISWC 2003. 2870:195-210.
2002
2002. Planning in a multi-agent environment: theory and practice. :944-945.
2002. HTN Planning in Answer Set Programming. UMIACS-TR-2002-18
2002. CaMeL: Learning method preconditions for HTN planning. :131-14.
2002. Theoretical and Empirical Aspects of a Planner in a Multi-agent Environment. Logics in Artificial Intelligence. 2424:173-185.
2002. M-Translog-2: A Planning Domain Designed for AIPS-2002. UMIACS-TR-2002-82
2002. On the Complexity of Plan Adaptation by Derivational Analogy in a Universal Classical Planning Framework. Advances in Case-Based Reasoning. 2416:199-206.
2001
2000
2000. Finding the maximal cutter for 2-D milling operations. :10-13.
2000. A Geometric Algorithm for Finding the Largest Milling Cutter. ISR; TR 2000-40
2000. A Geometric Algorithm for Multi-Part Milling Cutter Selection. ISR; TR 2000-42
2000. Selecting Flat End Mills for 2-1/2D Milling Operations. ISR; TR 2000-41
1998
1998. Feature Recognition for Interactive Applications: Exploiting Distributed Resources. UMIACS-TR-94-126.1
1998. IMACS: a case study in real-world planning. Intelligent Systems and their Applications, IEEE. 13(3):49-60.